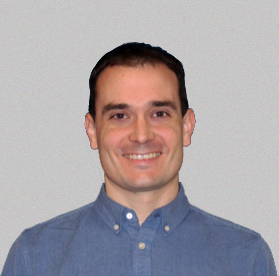
Hi. I'm Iñigo Urteaga.
I am a tenure-track LaCaixa Foundation Junior Leader and Ikerbasque Research Fellow in the Machine Learning group at the Basque Center for Applied Mathematics (BCAM). I have specialized in statistical machine learning, computational Bayesian statistics, approximate inference methods, and sequential decision processes.
I study statistical models and algorithms to extract information from data, for computer systems to effectively learn how to perform descriptive, predictive, and prescriptive tasks. My research is on methodological and applied aspects of probabilistic machine learning.
I was a Visiting Scholar at the Eric and Wendy Schmidt Center at the Broad Institute of MIT and Harvard in Fall 2024, and an Associate Research Scientist at Columbia University from 2018 to 2022, affiliated with its Applied Physics and Applied Mathematics department and Data Science Institute.
Previously, I was a data-science postdoctoral scientist at Columbia University from 2016 to 2018 working with Prof. Chris Wiggins and Prof. Noémie Elhadad on statistical machine learning for healthcare data, in the context of electronic health records and self-tracked data.
I completed my Ph.D. in Electrical Engineering at Stony Brook University in 2016 under the supervision of Prof. Petar M. Djurić. My dissertation is entitled "Sequential Monte Carlo methods for inference and prediction of time-series". My research attracted interest in econometrics (for prediction in stochastic volatility models) and biomedicine (for the study of fetal heart-rate signals).
I obtained my degree in telecommunications engineering from the UPV/EHU Faculty of Engineering in Bilbao, Spain, and worked in Research and Development projects at Tecnalia.